Machine Learning in Finance: A Comprehensive Overview
Machine learning (ML) has emerged as a transformative force across various industries, and the finance sector is no exception. By leveraging vast amounts of data and sophisticated algorithms, ML is revolutionizing financial processes, enhancing risk management, and driving innovation in product development and customer service. This comprehensive guide delves into the world of machine learning in finance, exploring its applications, benefits, challenges, and future implications.
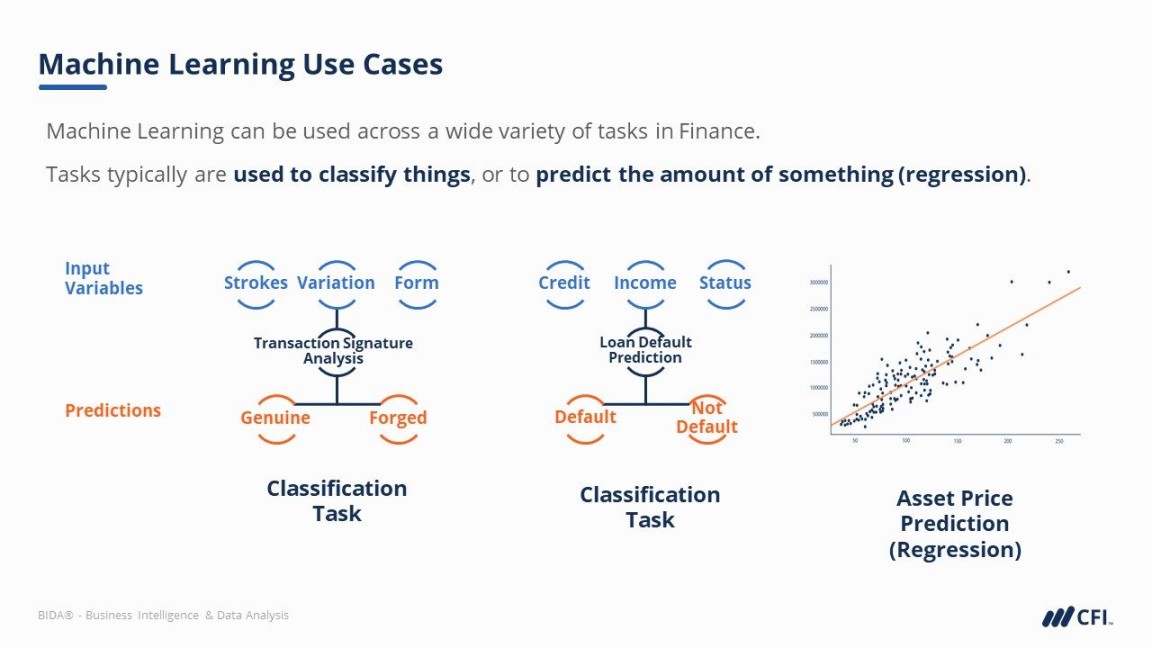
Machine learning in finance encompasses the application of ML techniques to analyze financial data, identify patterns, and make predictions or decisions. It empowers financial institutions to extract valuable insights from complex datasets, enabling them to automate tasks, optimize strategies, and gain a competitive edge.
ML algorithms in finance typically follow a structured approach:
1. Data Collection and Preprocessing: Gather relevant financial data from internal systems, external sources, and market feeds. Ensure data quality and consistency through preprocessing steps like cleaning, handling missing values, and transforming data into a suitable format.
2. Feature Engineering: Extract meaningful features from the raw data. This involves transforming, combining, and creating new variables that capture the underlying characteristics and patterns relevant to the financial problem being addressed.
3. Model Selection and Training: Choose an appropriate ML algorithm based on the task at hand. Train the selected algorithm using the prepared data, allowing it to learn from the patterns and relationships within the data.
4. Model Evaluation and Refinement: Evaluate the performance of the trained model on a separate validation dataset. Assess its accuracy, precision, and generalizability. Refine the model by adjusting parameters, adding features, or trying different algorithms if necessary.
5. Deployment and Monitoring: Deploy the validated model into production, integrating it into financial systems and processes. Continuously monitor the model’s performance and retrain it periodically with updated data to ensure its effectiveness over time.
Machine learning finds diverse applications across the financial spectrum, including:
1. Fraud Detection: Identify and prevent fraudulent transactions by analyzing historical patterns, customer behavior, and transaction characteristics.
2. Risk Management: Assess and manage credit, market, and operational risks by analyzing vast amounts of data and predicting potential risks.
3. Algorithmic Trading: Develop and implement trading strategies that use ML algorithms to analyze market data, identify trends, and execute trades automatically.
4. Customer Segmentation and Targeting: Segment customers based on their financial profiles, risk characteristics, and behavioral patterns. Tailor marketing campaigns and product offerings to specific customer segments.
5. Customer Churn Prediction: Identify customers at risk of churning and proactively address their concerns to improve customer retention.
The adoption of machine learning in finance brings several compelling benefits:
1. Enhanced Decision-Making: ML provides data-driven insights that inform better decision-making across various financial functions, leading to improved profitability, risk management, and customer satisfaction.
2. Automated Processes and Efficiency: ML automates repetitive and time-consuming tasks, freeing up staff to focus on higher-value activities and improving operational efficiency.
3. Personalized Customer Experiences: ML enables personalized financial products, services, and recommendations tailored to individual customer needs and preferences.
4. Risk Mitigation and Fraud Prevention: ML effectively identifies and prevents fraudulent activities, reducing financial losses and protecting customer data.
5. Market Trend Analysis and Prediction: ML algorithms can analyze vast amounts of market data to identify trends, predict future market movements, and inform investment decisions.
Despite its transformative potential, implementing machine learning in finance poses certain challenges:
1. Data Quality and Availability: Accessing and ensuring the quality, consistency, and relevance of large volumes of financial data is crucial for effective ML models.
2. Model Interpretability and Explainability: Understanding the rationale behind ML model decisions is critical for building trust and ensuring compliance with regulatory requirements.
3. Algorithmic Bias and Fairness: ML models must be carefully designed and trained to avoid biases that could lead to discriminatory or unfair outcomes.
4. Talent Acquisition and Retention: Recruiting and retaining skilled data scientists and ML engineers is essential for developing and maintaining sophisticated ML models.
5. Regulatory Compliance and Governance: Financial institutions must adhere to evolving data privacy regulations and ensure proper governance of ML models.
Machine learning is poised to play an even more prominent role in shaping the future of finance:
1. Personalized Financial Advice: ML will power personalized financial advice and wealth management solutions, tailored to individual risk profiles and investment goals.
2. Algorithmic Trading and Market Automation: ML-driven algorithmic trading will become more sophisticated, enabling faster, more efficient execution of complex trading strategies.
3. Regulatory Compliance and Risk Management: ML will enhance regulatory