Statistical Arbitrage Strategies: A Comprehensive Guide

Introduction
In the dynamic realm of finance, traders are constantly seeking ingenious methods to exploit market inefficiencies and generate profits. Among these strategies, statistical arbitrage has emerged as a compelling approach that utilizes sophisticated statistical techniques to identify and capitalize on pricing discrepancies across various financial instruments. This comprehensive guide delves into the intricacies of statistical arbitrage strategies, equipping readers with a thorough understanding of their concepts, applications, and potential benefits.
Understanding Statistical Arbitrage
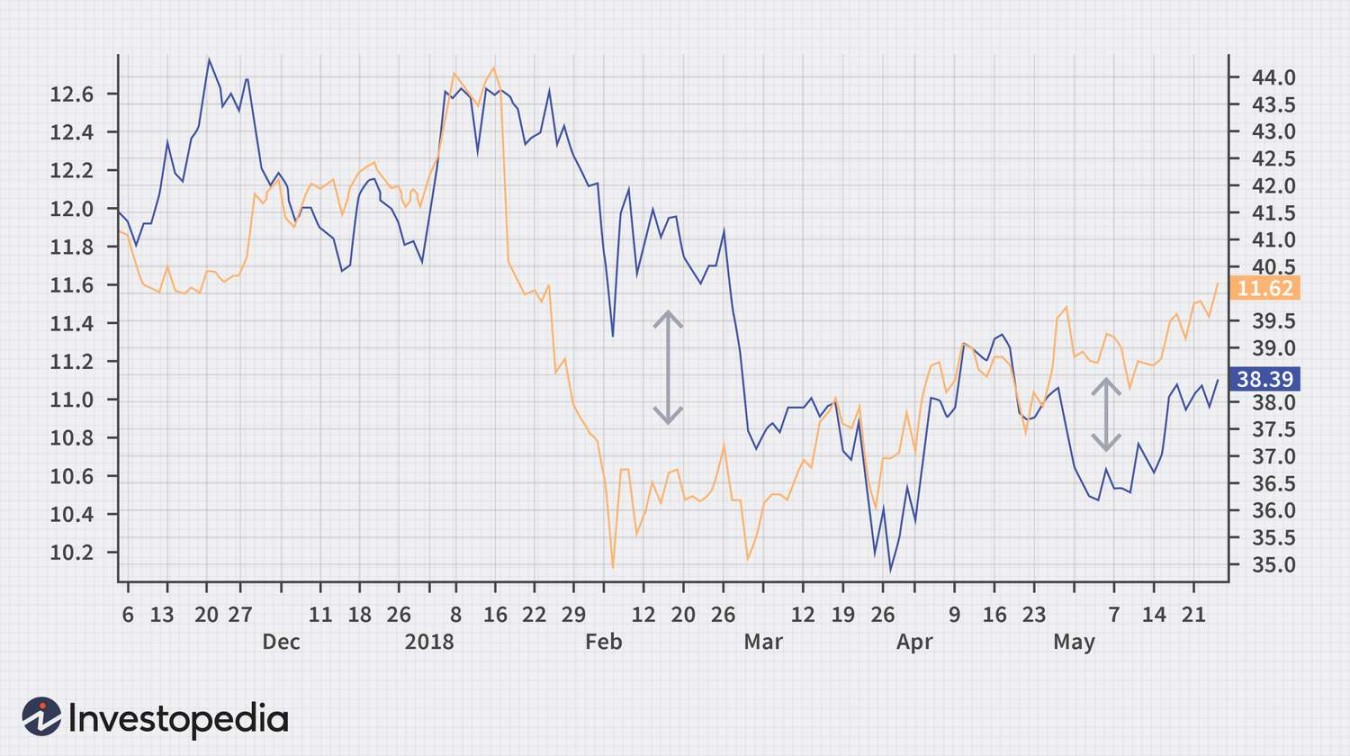
Statistical arbitrage, also known as stat arb or simply arb, is a quantitative trading strategy that aims to profit from mispriced securities by exploiting statistical anomalies in the market. These anomalies arise from inefficiencies or irrationalities in pricing, creating temporary imbalances that can be exploited by astute traders.
The core principle underlying statistical arbitrage lies in the identification of these pricing discrepancies. By employing sophisticated statistical models and algorithms, traders can scan vast amounts of market data to uncover these anomalies. Once identified, traders can execute arbitrage trades, simultaneously buying the undervalued security and selling the overvalued one, capitalizing on the price difference until the anomaly dissipates.
Key Characteristics of Statistical Arbitrage Strategies
Statistical arbitrage strategies are distinguished by several key characteristics that set them apart from traditional trading approaches:
1. Data-Driven Approach: Statistical arbitrage relies heavily on quantitative data analysis, employing complex statistical models and algorithms to identify pricing anomalies.
2. Emphasis on Efficiency: The strategy seeks to exploit market inefficiencies, capitalizing on pricing discrepancies that arise from irrationalities or market frictions.
3. Short-Term Focus: Statistical arbitrage trades typically involve short holding periods, aiming to profit from anomalies quickly before they disappear.
4. Scalability: The strategy can be scaled to accommodate large trading volumes, making it suitable for institutional investors and hedge funds.
Types of Statistical Arbitrage Strategies
Statistical arbitrage strategies can be broadly classified into two main categories:
1. Mean Reversion Strategies: These strategies focus on identifying securities that have deviated from their intrinsic value, betting on their prices to revert to the mean.
2. Pairs Trading Strategies: These strategies involve identifying pairs of securities that exhibit a historically stable price relationship but have temporarily diverged. The trader buys the undervalued security and sells the overvalued one, anticipating that the price relationship will normalize.
Implementation of Statistical Arbitrage Strategies
Implementing statistical arbitrage strategies involves a systematic process that encompasses several crucial steps:
1. Data Collection and Preprocessing: Gather and preprocess vast amounts of historical and real-time market data, ensuring data quality and consistency.
2. Model Development: Develop and refine statistical models and algorithms capable of identifying pricing anomalies and generating trading signals.
3. Risk Management: Implement robust risk management frameworks to mitigate potential losses and protect capital.
4. Execution and Monitoring: Execute trades efficiently and continuously monitor market conditions and model performance, making adjustments as needed.
Benefits of Statistical Arbitrage Strategies
Statistical arbitrage strategies offer several potential benefits to traders:
1. Potential for Consistent Returns: By exploiting market inefficiencies, these strategies can generate consistent returns over time.
2. Diversification Benefits: Statistical arbitrage strategies can provide diversification benefits, as they often have low correlation with traditional asset classes.
3. Scalability: The strategy can be scaled to accommodate large trading volumes, making it suitable for institutional investors.
Challenges of Statistical Arbitrage Strategies
Despite its potential advantages, statistical arbitrage also presents challenges that traders must consider:
1. Data Acquisition and Processing: Acquiring and processing large volumes of high-quality market data can be costly and time-consuming.
2. Model Complexity: Developing and maintaining sophisticated statistical models requires specialized expertise and computational resources.
3. Market Dynamics: Market conditions can change rapidly, requiring continuous model adaptation and risk management.
4. Competition: Statistical arbitrage is a competitive field, with numerous traders vying for the same opportunities.
Factors Affecting the Performance of Statistical Arbitrage Strategies
The performance of statistical arbitrage strategies is influenced by several factors:
1. Market Efficiency: The level of market efficiency can impact the availability and profitability of arbitrage opportunities.
2. Data Quality: The quality and timeliness of market data significantly impact the effectiveness of statistical models.
3. Model Sophistication: The sophistication and accuracy of statistical models are crucial for identifying true anomalies and generating profitable trades.
4. Execution Speed: The speed and efficiency of trade execution can influence overall profitability.
5. Risk Management: Effective risk management practices are essential to mitigate potential losses and protect capital.
Future of Statistical Arbitrage
As financial markets continue to evolve and technological advancements emerge, statistical arbitrage strategies are likely to undergo further refinement and adaptation.